Shapley Value
[[SHAP]](SHAPley Additive exPlanations)
计算例子:用年龄、性别、工作来预测收入
-
3 个特征能训练 8 个不同的模型
-
边际贡献:两个节点的预测之间的差距可以归因于附加特征的影响
-
下图中 w1 对应的边是该样本 age 带来的边际收益:50k - 40k = -10k
-
age 特征整体的计算公式为
-
-
S H A P_{\text {Age }}\left(x_0\right) & =w_1 \times \text { MCAge. }{\text { Age }}\left(x_0\right)+ \
& =w_2 \times \text { MCAge. }{\text { Age, Gender }}\left(x_0\right)+ \
& =w_3 \times \text { MCAge. }{\text { Age, Job }}\left(x_0\right)+ \
& =w_3 \times \text { MCAge. }{\text { Age, Gender }, \text { Job }}\left(x_0\right)
\end{aligned}
$A n d w_1+w_2+w_3+w_4=1$ + 权重 w 可以设定为同一行中边总数m的倒数 $\frac{1}{m}$ + 最终计算公式为: 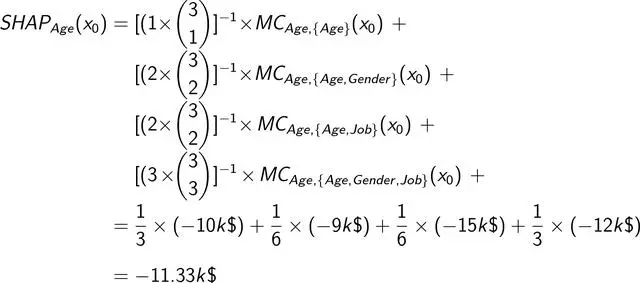 ## Ref + [理解用于计算SHAP值的公式 (baidu.com)](https://baijiahao.baidu.com/s?id=1654791131903418801&wfr=spider&for=pc)